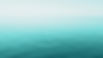
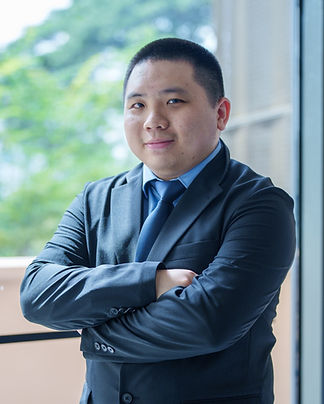
Xu Yuecong, PhD
Lecturer @ ECE Department, College of Design and Engineering, NUS, Singapore
​
yc.xu at nus.edu.sg; xuyu0014 at e.ntu.edu.sg
-
I am currently a Lecturer at the ECE department of the College of Design and Engineering (CDE), National University of Singapore (NUS). My current courses cover Computer Vision and Machine (Deep) Learning.
-
I obtained my Ph. D. Degree from Nanyang Technological University, Singapore, under the supervision of Prof. Mao Kezhi in 2021. My research focuses on video analytics and time-series analytics, with a special interest in applying transfer learning towards human action recognition as well as AIOT tasks. Previously, I obtained my Bachelor's Degree from NTU Singapore.
-
I was a Research Scientist at the Institute for Infocomm Research (I2R), Agency for Science, Technology, and Research (A*STAR) Singapore, from July 2021 to January 2024.
-
I was also a Part-Time Lecturer at the School of EEE, NTU Singapore from July 2021 to January 2024. My courses covered Computer Vision and Machine Learning.
Recent News
-
[2024/02] Track 5 of the 7th UG2+ Workshop (in conjunction with CVPR 2024) is now officially launched. Call for participation and details of competition are available [Here]! We look forward to your participation!
-
[2024/01] I am appointed as the Young Associate Editor for Journal of Automation and Intelligence.
-
[2024/01] One paper has been accepted by ICRA!! Congrats to all the collaborators!! The ArXiv version is available [Here]!
-
[2024/01] One paper has been accepted by ICLR!! Congrats to all the collaborators!! Check our ArXiv version and the project page [Here]!
-
[2024/01] I am joining NUS as a lecturer (Educator Track) with the ECE department, CDE. Course information for Semester 2 AY 23/24 will be available [Here].
-
[2023/12] Two papers have been accepted by AAAI!! Congrats to all the collaborators!! ArXiv versions are available [Here] and [Here]. See you all in Vancouver!
-
[2023/09] One paper has been accepted by NeurIPS Datasets and Benchmarks Track!! Congrats to all the collaborators!! Click [Here] for more details.
-
[2023/06] Two papers have been accepted by ICCV (Both as (co-) first author!)!! Click [Here] and [Here] for more details.
-
[2023/03] One paper has been accepted by IEEE T-Cyber!! Click [Here] for more details. The public ArXiv version can be found [Here].
-
[2023/01] One paper has been accepted by IEEE TCSVT!! Click [Here] for more details. The published version can be found [Here].
-
[2022/11] One paper has been accepted by AAAI!! Click [Here] for more details.
-
[2022/11] Our survey on video unsupervised domain adaptation (VUDA) is now on ArXiv. The corresponding GitHub repository is also available. Click [Here] for details.
-
[2022/10] One paper has been accepted and published by IEEE TNNLS!! Click [Here] for more details. The published version can be found [Here].
-
[2022/07] One paper has been accepted and published by ECCV 2022!! Click [Here] for more details.
-
[2022/07] One paper has been accepted and published by ACMMM 2022!! Click [Here] for more details.
-
[2022/06] The 5th UG2+ Workshop, was held in conjunction with CVPR2022 in hybrid mode. The result of the UG2+ 2022 Challenge is available, congratulations to all winners!
-
[2022/02] I am invited as the Session Chair for ICARCV 2022.
-
[2021/09] One paper has been accepted and published by ICCV 2021 as Oral Paper!!. Click [Here] for more details.
-
[2021/06] The 4th UG2+ Workshop, was held in conjunction with CVPR2021. We thank all participants for their participation and full support.
-
[2020/06] We publish ARID Dataset! Sample code available now, click [Here]
Research Interests
My research mainly focuses on Video Analytics, with a special interest in Video-based Action Recognition, where state-of-the-art AI models are proposed to analyze daily actions for applications such as security, smart-home and autonomous control. More specifically, I study how AI models are efficiently deployed in resource-restrained conditions and how multimodal data from different sensors could aid accurate video analysis. To achieve the above goals, I and my team mainly touch on the following topics:
-
Video Analysis (Action Recognition) with Less Data: To improve the performance of video-based tasks (e.g., action recognition), various deep models have been proposed. The success of such deep models relies heavily on the availability of large-scale labeled datasets, which are built to contain as many scenarios as possible. However, this may not be possible in various conditions, for example, when data privacy is an issue, or in adverse environments where video data are not available. Meanwhile, training on large-scale datasets is both resource and time-consuming and is not end-user-friendly. AI models should break away from the need for large-scale labeled datasets, which denotes our objective. We develop cutting-edge AI models with transfer learning where we utilize less data in adverse or privacy concerned scenarios while maintaining the performance of AI models.
​
-
Multimodal Learning for Robust Video Analysis: While analyzing videos based on the visual modal is the main-stream solution, visual models may not work well under certain conditions such as adverse illumination or adverse contrast. This may hamper the deployment of visual models in real-world applications such as autonomous driving, where robust video analysis is vital for precise decision-making. In these conditions, sensors such as WiFi or radar/lidar could potentially aid visual models. To leverage the complementarity of the various modalities, we study on potential multimodal algorithms for robust video analysis under all kinds of environments.